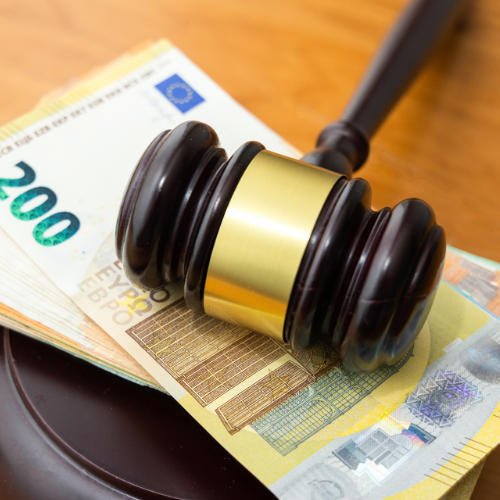
Danske Commodities & Equinor fined EUR 8 Million for Gas Capacity Market Manipulation
The French Energy Regulatory Commission (CRE) fined Danske Commodities A/S and Equinor ASA €8M for gas capacity auction market manipulation.
The article discusses a study by the Netherlands Authority for Consumers and Markets (ACM) on algorithmic trading in wholesale energy markets, focusing on trends, impacts, and compliance. It also reviews the Futures Industry Association (FIA) report, which offers best practices for mitigating risks in automated trading, with guidance applicable to energy and commodity trading firms.
This analysis is crucial because it highlights the growing influence of algorithmic trading in energy markets, emphasizing the need for robust compliance and risk management to ensure market integrity. The insights from ACM and FIA reports guide firms in aligning with regulatory standards and mitigating risks, making them essential for maintaining transparent and efficient trading environments.
Key takeaways include the ACM's findings on the types and impacts of algorithms in energy trading, emphasizing the balance between efficiency and risks like volatility and transparency. The FIA's best practices offer a framework for pre- and post-trade controls to manage these risks effectively. The importance of stringent compliance with regulations like REMIT 2 and MiFID 2 is underscored to maintain market integrity.
The Netherlands Authority for Consumers and Markets (ACM) has conducted a comprehensive study on algorithmic trading in wholesale energy markets (click here). The study, in collaboration with the Dutch Authority for Financial Markets (AFM), aimed to understand the trends, types, motives, impacts, and compliance procedures related to algorithmic trading in the wholesale energy sector.
In addition to ACM’s market study, the Futures Industry Association (FIA) has published a report entitled ‘Best Practices For Automated Trading Risk Controls And System Safeguards’ (click here) which provides insights into best practice risk controls used by firms and exchanges to govern automated trading activity .
Both reports provide welcomed insights into algo trading practices with the ACM study focused solely on wholesale energy markets while the FIA report provides broader risk control frameworks for governing automated trading activity.
ACM market study overview
The market study is the first of its kind issued by a National Regulatory Authority (NRA) specifically on algo trading in wholesale energy markets and provides insights across a variety of themes including:
The report outlines both the opportunities and risks associated with algorithmic trading while providing compliance guidance to market participants when applying governance over algo trading with a specific emphasis on REMIT 2 and MiFID 2.
Manon Leijten, Member of the Board of ACM, commented on the report noting:
"ACM enforces compliance with the rules regarding transparency and integrity of energy markets. This also applies to the use of algorithms in energy trading. It offers opportunities for, for example, the energy transition, but we are also aware of the risks. It is important that wholesale energy markets function well, and prices are formed in a fair manner. In that effort, we work together with the AFM. The insights gained through this study help us organize our oversight more effectively."
REMIT 2 vs. MiFID 2. While the focus on the algo trading compliance elements in the report is primarily on REMIT 2 requirements, the report concludes by sharing parallels to MiFID 2 rules and market participants' concerns over algo trading obligations under REMIT 2.
The survey notes that some market participants aligned their algo governance framework to MiFID 2 RTS 6 standards and thus do not expect much change with the introduction of REMIT 2 algo trading obligations. However, some market participants have expressed worries or uncertainties regarding the requirements on algorithmic trading introduced by the revised REMIT and seek further clarification or explanation on, for instance, technical standards to enhance their comprehension and implementation of the new obligations.
It is commendable that the ACM, a regulator responsible for overseeing such a small portion of the EU wholesale energy market, has taken the initiative to provide market participants with such valuable insights and perspectives into this critical topic. One might hope that this sets an example for the larger NRA's to follow suit.
Firms either exploring the implementation of algo trading strategies or who are currently conducting algo trading activity are strongly advised to read the 44-page report in detail.
FIA report overview
The Futures Industry Association (FIA) has developed a comprehensive set of best practices aimed at mitigating the risks associated with automated trading. The report provides an update to best practices for automated trading risk controls and system safeguards for trading firms, brokers, and exchanges.
For energy and commodity firms who currently engage in algo trading activity or who are exploring algo trading as a new strategy, the FIA report provides best practice governance frameworks for both pre-trade and post-trade controls that can be benchmarked, and where appropriate, included in your firms’ overall risk framework.
Below is a high-level summary of pre and post-trade controls provided in the report and which can be benchmarked against current algo trading risk governance procedures.
Pre-Trade Controls
Pre-trade controls are critical in preventing unauthorized market activity due to system failures, errors, or unauthorized access. Key pre-trade controls include:
Post-Trade Controls
Post-trade analysis involves a combination of monitoring, data collection, and controls to watch for potential credit events or unintended trading. Key post-trade controls include:
We review the ACM report in further detail below.
The ACM report provides a comprehensive overview of algo trading evolution specifically on wholesale energy market as noted above across the following four themes:
[1] Prevalence and Types of Algorithms
The report provides visual representations of the different types of algos being used (see page 17) and breaks down how each type of algo is being applied in the power and gas markets. The three types of algos currently in use are:
ACM analysed how algorithmic trading is used in both spot power markets and natural gas markets across the three types of algorithms:
"The results of the survey indicate that, in the natural-gas market execution algorithms are more frequently used than signal generators and trading algorithms, while, in the power market, all types of algorithms are used to the same extent."
Natural Gas Trading. Out of a total of 8 respondents that use and/or develop algorithms for gas trading:
Spot Power Market Trading. Approximately nine out of 12 respondents use and/or develop algorithms for power trading.
[2] Drivers of Algorithmic Trading
The shift towards renewable energy sources and the need for efficient trading processes are key drivers behind the increasing use of algorithms in energy markets. Algorithms help market participants manage the complexities of trading renewable energy, which requires constant balancing due to the unpredictable nature of production from sources like wind and solar.
That being said, in certain markets, trading without algorithms is becoming increasingly difficult, due to some disadvantages related to the slower speed of manual trading.
[3] Market Impact of Algorithmic Trading on Wholesale Energy Markets
ACM continues to monitor algo trading activity and notes that there are both positive outcomes and risks associated with algorithmic trading. Algorithmic trading has a significant impact on market dynamics, particularly in terms of liquidity and price formation. While algorithms enhance market efficiency and reduce trading costs, they also introduce challenges such as increased volatility and potential disconnects between market fundamentals and trading behaviour.
Positive Outcomes
Risks and Challenge
[4] Compliance and Regulation (REMIT & MiFID 2)
ACM stresses the importance of compliance and internal checks by firms as algo trading becomes more prevalent in energy markets, and reminds market participants that they must ensure that their trading activities need to adhere to regulatory standards to maintain market integrity and transparency, specifically the recently updated REMIT 2 regulation which came into effect in May 2024.
It also outlines market manipulation use cases in algo trading and the changes to surveillance methods required to monitor algo trading.
Market Manipulation in Algo Trading – ‘Robot Battles’ & Order Activity Disruption
ACM notes that businesses need to prevent the use of algorithms from resulting in illegal trading activities, such as market manipulation. Two examples of market manipulation include [a] Robot Battles and [b] Order Activity Disruption.
[a] Robot Battles
‘Robot Battles’ is where two algorithms end up bidding against each other through a series of order adjustments until one algo hits its price limit. ACM provides a description of this behaviour in further detail:
"In this scenario, two algorithms compete with each other for the best (highest) buy order through a series of order adjustments. This competition continues until the algorithm representing the ‘suspect’ party reaches its price limit. At this point, the party sells at the highest priced buy order of the other party and swiftly removes its own buy order. The concern would be that the purchase price is being manipulated, given that it is pushed up to its maximum limit through layering / spoofing behaviour, which involves the rapid removal of an order on one side of the book after a transaction on the opposite side."
[b] Order activity is so extreme that it disrupts the visibility of the order book for other market participants.
Algo improvement loops is where two or more algos compete to optimise their orders. In certain scenarios, the rapid adjustments e.g. thousands of adjustments per minute can lead to a scenario where the order book is no longer visible for other traders. ACM provides a further explanation:
"This situation can arise from the correlation between algorithms that leads to improvement loops or feedback loops. These loops manifest through upward and downward order price movements, where two or more algorithms compete to optimise their orders."
Imagine a situation where two algorithms compete to be the best (lowest) sell order in the order book. When algorithm ‘A’ adjusts its price to a level that is below the price limits of algorithm ‘B’, then algorithm ‘B’ no longer alters its price such that it is lower that algorithm ‘A’. Instead, algorithm ‘B’ changes its price to be the second-best price in the order book, just slightly better than the former second-best order in the order book. Subsequently, algorithm ‘A’ follows suit by adjusting its price which slightly betters the new second-best price in the order book of algorithm ‘B’. With the best price (of algorithm ‘A’) again being within the price limits of algorithm ‘B’, algorithm ‘B’ then pursues to have the best price again by slightly bettering the price of algorithm ‘A’.
These price adjustments continue, leading to upward and downward movements. The duration of such patterns depends on the programming of the algorithms. In specific circumstances, very rapid adjustments (imagine thousands of adjustments per minute) might lead to a situation where the order book is no longer visible for other traders.
Surveillance methods to Monitor Algo Trading
The ACM observed that the increased use of trading algorithms has led to changes in surveillance methods. Specifically, investigations into suspicious algorithmic trading behaviours now demands a more data-intensive approach compared with manual trading with a shift towards quantitative data analysis and the development of advanced detection tools. ACM notes that regulators are investing in expertise and IT infrastructure to enhance their ability to detect and analyse such trading behaviours.
Compliance and Internal Checks – Measures Taken By Market Participants
ACM notes that effective compliance and internal controls are essential for managing the risks associated with algo trading. They observed that market participants have developed a range of measures to ensure their algorithms perform as intended and comply with regulatory requirements as follows:
In addition to the above observations, ACM provides further detail on governance over specific components of algo trading.
"Most of the surveyed and interviewed market participants, who currently use algorithms or who are developing algorithms, apply many pre-trade limits. Based on the survey, the following pre-trade limits are used (ordered by descending use intensity):
"The majority of the surveyed market participants who currently use algorithms or who are developing algorithms have an automated surveillance system in place (12 out of 15) and require the trader to always monitor the orders of the algorithm (12 out of 15). This also holds for the interviewed market participants. Generally, such a monitoring system generates alerts for several types of problems."
"Compliance measures minimise the occurrence of adverse behaviour, though some factors may still possibly lead to unwanted outcome.
REMIT Legislative Developments in the EU Wholesale Energy Market
The Regulation on Wholesale Energy Market Integrity and Transparency (REMIT) has been revised (i.e. REMIT 2) to address the evolving landscape of algorithmic trading. These revisions introduce new obligations for market participants and enhance the regulatory oversight capabilities of authorities like ACM.
ACM provides an overview and reminder of new REMIT obligations for market participants engaged in algorithmic trading as follows:
In addition, ACM provides an overview of its enhanced oversight and responsibilities for monitoring of algo trading alongside the Dutch financial regulator AFM as follows:
ACM concludes its report noting future focus areas for additional market research studies as follows: